F1 Ratings: Understanding The Metrics
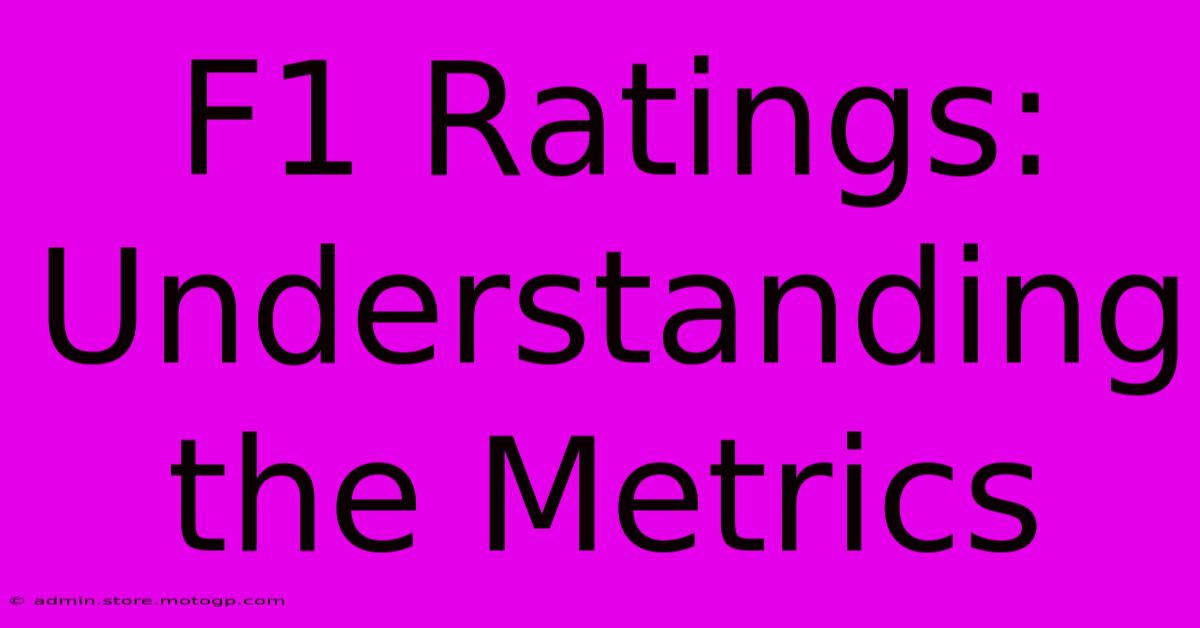
Table of Contents
F1 Ratings: Understanding the Metrics and How They Impact Your Business
Formula 1 racing is captivating, but understanding F1 ratings in the context of machine learning and information retrieval is equally important. This metric plays a crucial role in assessing the performance of a classification model, particularly in situations with imbalanced datasets. Let's delve into what F1 score truly means and its significance.
What is the F1 Score?
The F1 score is a crucial metric for evaluating the performance of a classification model. It's the harmonic mean of precision and recall. Unlike the simple average, the harmonic mean gives more weight to lower values, meaning a model needs to perform well on both precision and recall to achieve a high F1 score. This is particularly important when dealing with imbalanced datasets, where a high accuracy score might be misleading.
Understanding Precision and Recall
Before diving deeper into the F1 score, let's clarify its constituent parts:
-
Precision: Out of all the instances predicted as positive, what proportion was actually positive? It answers the question: "Of all the items we predicted as positive, how many were actually positive?" High precision indicates fewer false positives.
-
Recall (Sensitivity): Out of all the actual positive instances, what proportion did the model correctly identify? It answers the question: "Of all the items that were actually positive, how many did we correctly identify?" High recall indicates fewer false negatives.
Calculating the F1 Score
The F1 score is calculated using the following formula:
F1 = 2 * (Precision * Recall) / (Precision + Recall)
A perfect F1 score is 1, while a score of 0 indicates poor performance.
Why is the F1 Score Important?
The F1 score provides a balanced measure of a classifier's performance. It's especially valuable when:
-
The dataset is imbalanced: In scenarios with significantly more negative instances than positive ones (or vice-versa), accuracy alone can be deceptive. A model might achieve high accuracy by simply classifying everything as the majority class. The F1 score, however, penalizes such behavior.
-
Both precision and recall are crucial: Imagine a spam detection system. A high recall is crucial to avoid missing actual spam emails (false negatives). However, high precision is also important to avoid classifying legitimate emails as spam (false positives). The F1 score balances these competing needs.
-
You need a single metric to compare models: When comparing multiple models, the F1 score offers a concise and informative single number summarizing performance.
F1 Score vs. Other Metrics
While accuracy is a commonly used metric, the F1 score provides a more nuanced view of performance, especially in imbalanced scenarios. Other metrics, such as the area under the ROC curve (AUC-ROC), also offer valuable insights. However, the F1 score's simplicity and direct interpretation make it a favored choice in many applications.
Interpreting F1 Scores
The interpretation of the F1 score depends on the context. A high F1 score (close to 1) indicates excellent performance; a low score suggests the need for model improvement. The specific threshold for "good" or "bad" varies based on the application and acceptable levels of false positives and false negatives.
Optimizing for F1 Score
Improving the F1 score often involves techniques such as:
- Data augmentation: Increasing the number of samples, particularly in the minority class.
- Resampling techniques: Using methods like oversampling the minority class or undersampling the majority class.
- Adjusting the classification threshold: This impacts the balance between precision and recall.
- Feature engineering: Selecting or creating more informative features.
- Trying different algorithms: Exploring various classification algorithms (e.g., Random Forest, Support Vector Machines, Gradient Boosting) to find the best fit for your data.
Conclusion
The F1 score is a powerful tool for evaluating classification models, particularly in real-world applications where both precision and recall are crucial. By understanding its calculation and implications, you can effectively assess model performance and make informed decisions about model selection and improvement. Remember to always consider the specific context of your application when interpreting the F1 score. It's a crucial metric for building robust and reliable machine learning systems.
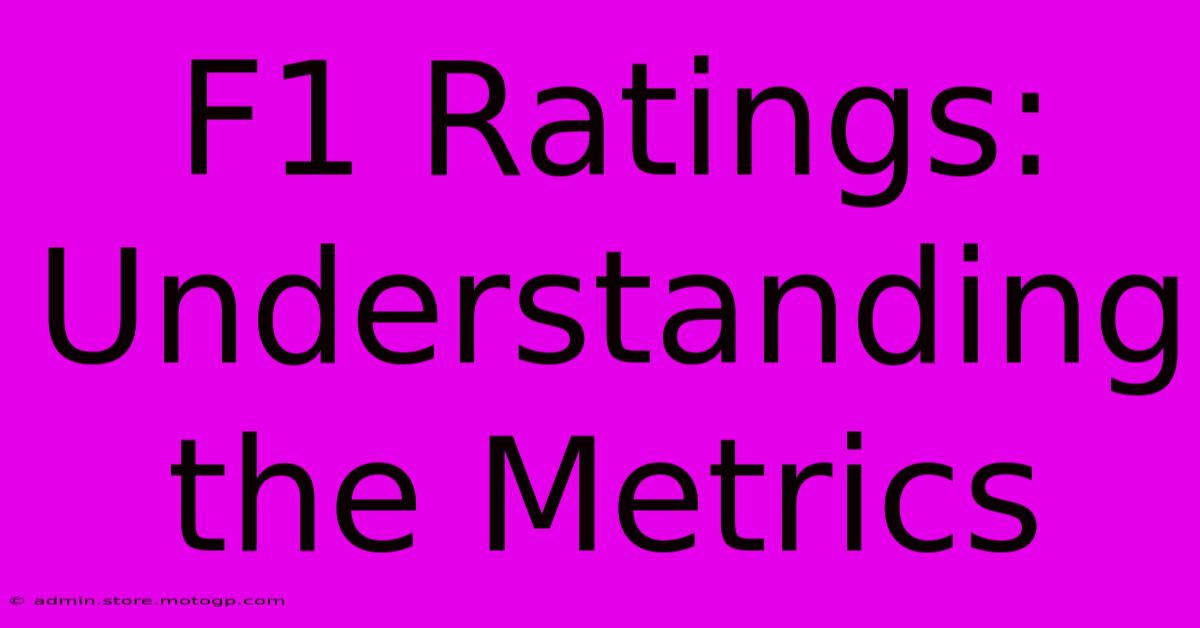
Thank you for visiting our website wich cover about F1 Ratings: Understanding The Metrics. We hope the information provided has been useful to you. Feel free to contact us if you have any questions or need further assistance. See you next time and dont miss to bookmark.
Featured Posts
-
Lot F Cota A Game Changer
Feb 19, 2025
-
F1 Shuttle Your Ultimate Race Day Experience
Feb 19, 2025
-
F1 Event Houston Where Passion Meets Performance
Feb 19, 2025
-
Moto 2 Bike Specs Master The Art Of Moto 2 Racing With These Specs
Feb 19, 2025
-
Circuit Of The Americas Hospitality Designed For You
Feb 19, 2025