From Correlation To Causation? The Power Of PMI
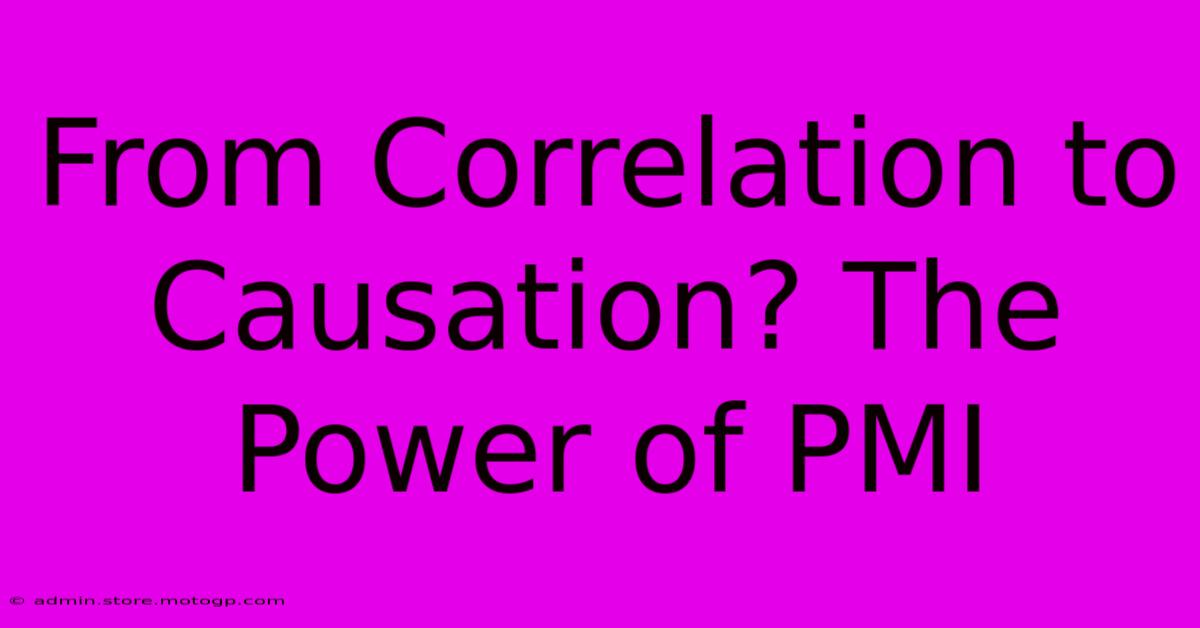
Table of Contents
From Correlation to Causation? The Power of PMI
Understanding the relationship between variables is crucial in many fields, from business analytics to scientific research. Often, we start by observing correlations – two things happening together. But correlation doesn't equal causation. Just because two things are linked doesn't mean one causes the other. This is where PMI, or Pointwise Mutual Information, comes in. This powerful statistical measure helps us move beyond simple correlation and delve deeper into the potential causal links between variables.
What is Pointwise Mutual Information (PMI)?
PMI quantifies the amount of information one random variable tells us about another. In simpler terms, it measures how much knowing the value of one variable helps us predict the value of another. A high PMI suggests a strong relationship, hinting at a possible causal connection (though it doesn't definitively prove it). A low PMI indicates a weak or nonexistent relationship.
How does it work? PMI is calculated using probabilities. It compares the joint probability of two events occurring together to the product of their individual probabilities. A formula looks like this:
PMI(X, Y) = log₂ [P(X, Y) / (P(X) * P(Y))]
Where:
P(X, Y)
is the probability of both X and Y occurring together.P(X)
is the probability of X occurring.P(Y)
is the probability of Y occurring.
A positive PMI value indicates a positive relationship – the events tend to occur together more often than expected by chance. A negative PMI value suggests a negative relationship – they occur together less often than expected. A PMI of zero means the events are independent; knowing one tells us nothing about the other.
PMI in Action: Real-World Examples
Let's imagine we're analyzing website traffic. We might find a correlation between the number of social media shares and website visits. But is one causing the other? PMI can help answer this. By calculating the PMI between "social media shares" and "website visits," we can quantify the strength of their relationship and gain insight into whether increased social media activity predicts higher website traffic.
Other examples of PMI applications include:
- Natural Language Processing (NLP): Identifying word collocations and relationships between words in text corpora.
- Bioinformatics: Analyzing gene expression data to understand gene interactions.
- Market Research: Understanding consumer behavior and preferences.
- Recommendation Systems: Predicting user preferences based on past actions.
Moving Beyond Correlation: The Importance of Context
While PMI is a powerful tool, it's crucial to remember that correlation does not equal causation. A high PMI suggests a strong relationship, increasing the likelihood of a causal link, but it doesn't definitively prove it. Other factors could be involved, creating a spurious correlation.
To strengthen the case for causation, we need to consider other factors:
- Temporal Precedence: Does the presumed cause precede the effect in time?
- Alternative Explanations: Are there other variables that could explain the observed relationship?
- Mechanism: Is there a plausible mechanism that explains how the cause leads to the effect?
By combining PMI with other analytical techniques and careful consideration of context, we can move from identifying correlations to building a stronger case for causation.
Conclusion: Utilizing PMI for Deeper Insights
PMI is a valuable statistical measure for exploring the relationships between variables. It allows us to move beyond simple correlations and quantify the strength of those relationships, providing clues about potential causal links. However, it's essential to remember that PMI alone cannot prove causation. By using PMI in conjunction with other analytical techniques and a thorough understanding of the context, we can gain deeper insights into complex systems and make more informed decisions. This sophisticated approach enables us to move from mere observation to a more nuanced understanding of cause and effect.
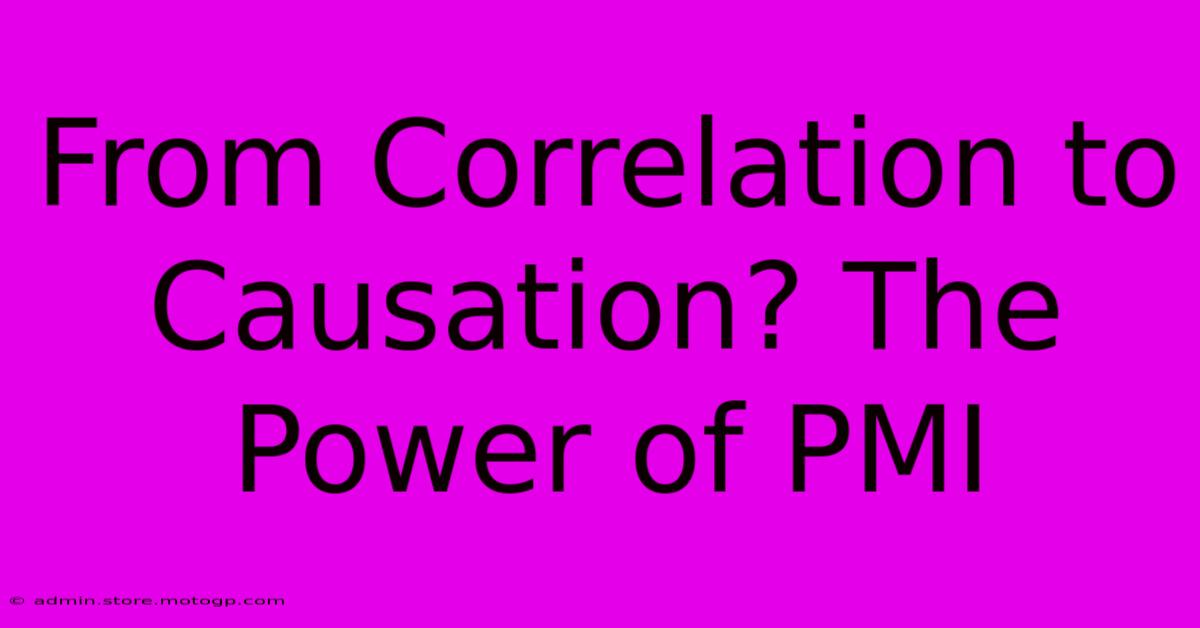
Thank you for visiting our website wich cover about From Correlation To Causation? The Power Of PMI. We hope the information provided has been useful to you. Feel free to contact us if you have any questions or need further assistance. See you next time and dont miss to bookmark.
Featured Posts
-
Machu Picchu Conquering Altitude For An Unforgettable Experience
Feb 12, 2025
-
Experience The Majesty Cathedral Of St Matthew The Apostle
Feb 12, 2025
-
New Yorks Dobbs Ferry Small Town Feel Big City Perks
Feb 12, 2025
-
1922 Silver Dollar Unveiling Its True Worth
Feb 12, 2025
-
Lewis Vs Tyson Finally Settled
Feb 12, 2025