GMM: Unbiased Insights, Even With Imperfect Data
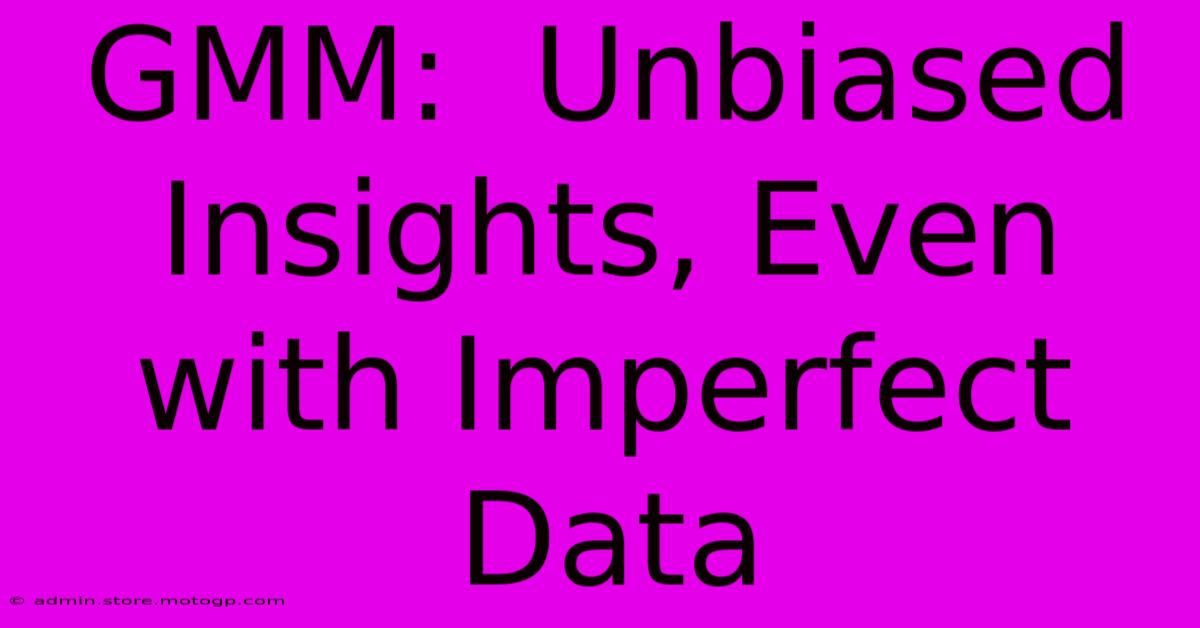
Table of Contents
GMM: Unbiased Insights, Even with Imperfect Data
Gaussian Mixture Models (GMMs) are powerful statistical tools used for clustering and density estimation. Unlike some methods that falter in the face of noisy or incomplete data, GMMs offer a robust approach to uncovering hidden patterns, even when the data isn't perfect. This article explores the strengths of GMMs in handling imperfect data and demonstrates their practical applications.
What is a Gaussian Mixture Model (GMM)?
At its core, a GMM assumes that the data points are generated from a mixture of several Gaussian distributions (normal distributions). Each Gaussian represents a different cluster or subgroup within the data, characterized by its own mean (center) and covariance matrix (shape and spread). The model estimates the parameters of these Gaussians – their means, covariances, and mixing proportions (the probability of a data point belonging to each cluster) – to best fit the observed data.
Why GMMs Excel with Imperfect Data
Several factors contribute to GMM's robustness when dealing with noisy or incomplete datasets:
-
Probabilistic Nature: GMMs work with probabilities, not hard assignments. Each data point is assigned a probability of belonging to each cluster, acknowledging the inherent uncertainty, especially when the data is noisy or overlapping. This is unlike k-means clustering, which assigns points to a single cluster definitively.
-
Handling Missing Data: GMMs can be adapted to handle missing data points. Expectation-Maximization (EM) algorithms, commonly used to fit GMMs, can be modified to effectively incorporate incomplete data during the parameter estimation process. The algorithm iteratively estimates missing values and model parameters until convergence.
-
Flexibility in Modeling Complex Distributions: The ability to model data using multiple Gaussian components allows GMMs to capture complex, non-spherical data distributions, which often arise from real-world, imperfect datasets. A single Gaussian wouldn't suffice to model such intricate structures.
Applications of GMMs with Imperfect Data
The robustness of GMMs makes them suitable for a wide range of applications where data quality might be compromised:
-
Image Segmentation: GMMs can effectively segment images even with noise or artifacts, separating different regions based on color or texture characteristics. Missing pixels due to image corruption are handled gracefully.
-
Anomaly Detection: By modeling the "normal" behavior of a system using a GMM, anomalies that deviate significantly from the learned distribution can be identified. This is particularly valuable in scenarios where some data points are inherently noisy or erroneous.
-
Financial Modeling: In finance, GMMs can be employed to model the distribution of asset returns, even when the data is subject to market noise or measurement error. This can inform risk assessment and portfolio optimization strategies.
-
Speech Recognition: GMMs are frequently utilized in speech recognition systems to model the acoustic characteristics of different phonemes (speech sounds). These models demonstrate robustness to variations in speaker's voice and background noise.
-
Customer Segmentation: In marketing, GMMs help to segment customers into distinct groups based on their purchasing behavior, even with incomplete or noisy customer data. This supports targeted marketing and product development efforts.
Limitations and Considerations
While GMMs offer significant advantages, it's crucial to acknowledge some limitations:
-
Computational Cost: Fitting GMMs, particularly for high-dimensional data or a large number of clusters, can be computationally expensive.
-
Sensitivity to Initialization: The EM algorithm used to fit GMMs can converge to local optima, meaning the results might depend on the initial parameter values. Multiple runs with different initializations are often recommended.
-
Model Selection: Choosing the optimal number of Gaussian components is crucial. Various model selection criteria, such as the Bayesian Information Criterion (BIC), can assist in determining the appropriate model complexity.
Conclusion: GMMs – A Powerful Tool for Real-World Data Analysis
Gaussian Mixture Models offer a robust and flexible approach to data analysis, even when dealing with imperfect data. Their probabilistic nature, ability to handle missing data, and capacity to model complex distributions make them invaluable tools across various domains. While computational cost and sensitivity to initialization are considerations, the strengths of GMMs often outweigh these limitations, providing unbiased insights from real-world data, where imperfections are often the rule, rather than the exception. By understanding their capabilities and limitations, you can effectively leverage GMMs to uncover meaningful patterns and derive actionable conclusions from your datasets.
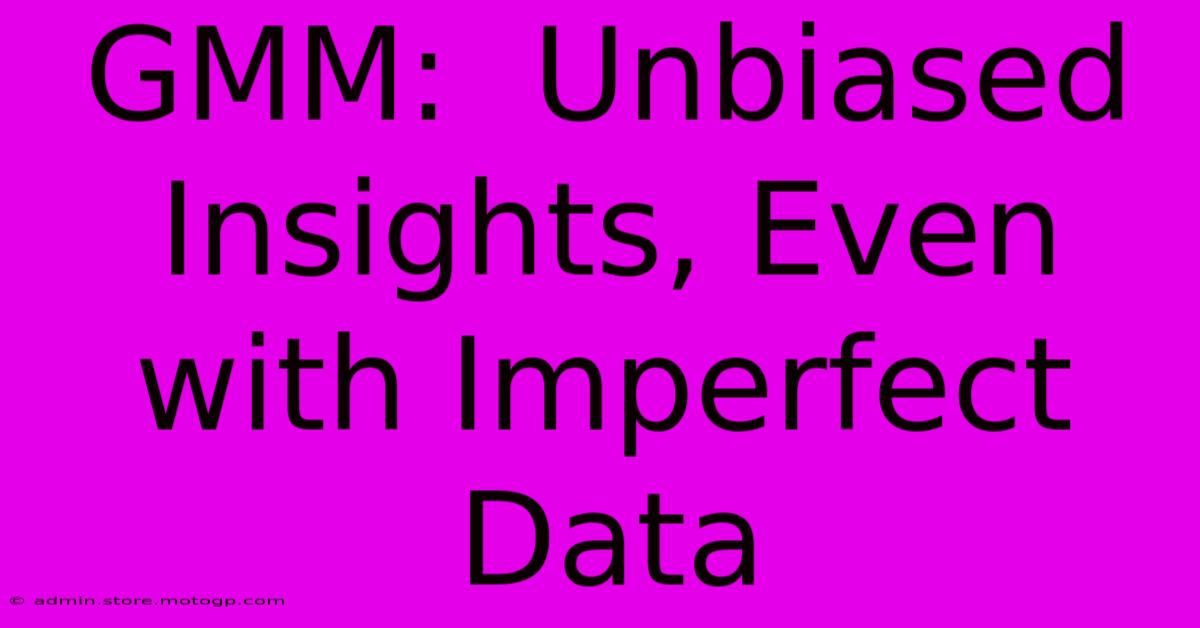
Thank you for visiting our website wich cover about GMM: Unbiased Insights, Even With Imperfect Data. We hope the information provided has been useful to you. Feel free to contact us if you have any questions or need further assistance. See you next time and dont miss to bookmark.
Featured Posts
-
Chile Vs Argentina Whos On Top
Feb 11, 2025
-
The Tower Of Babels Height Debunking The Myths
Feb 11, 2025
-
Movie Night Sorted Naayak And Mirchi Dvds Available
Feb 11, 2025
-
The Secret To Using Sesac Music Without Issues
Feb 11, 2025
-
Stress Free Parenting Learn From Franklins Mistakes
Feb 11, 2025