The Positive Definite Matrix: A Key To Robust Solutions
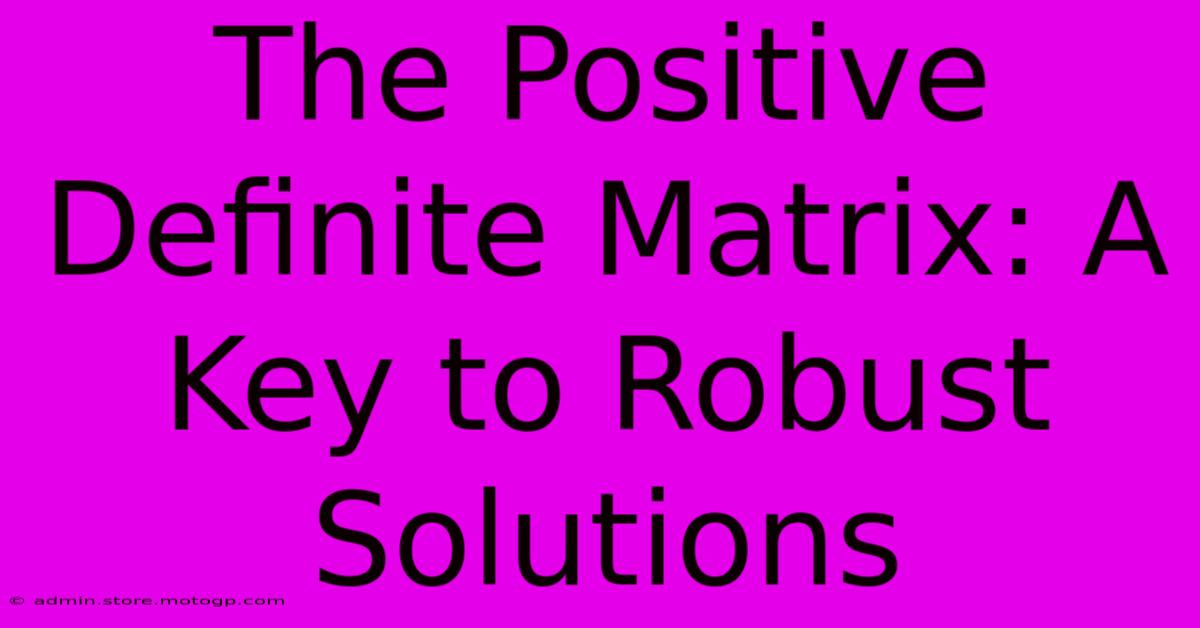
Table of Contents
The Positive Definite Matrix: A Key to Robust Solutions
Positive definite matrices are far more than just a theoretical concept in linear algebra; they are fundamental building blocks for robust and reliable solutions across numerous fields. Understanding their properties is crucial for anyone working with optimization, machine learning, statistics, and even physics. This article delves into the world of positive definite matrices, exploring their definition, properties, and crucial applications.
What is a Positive Definite Matrix?
A positive definite matrix is a symmetric matrix where all its eigenvalues are positive. Alternatively, a symmetric n x n real matrix A is positive definite if and only if x<sup>T</sup>Ax > 0 for all non-zero vectors x in R<sup>n</sup>. This seemingly simple definition hides a wealth of implications. The condition x<sup>T</sup>Ax represents a quadratic form, and its positivity ensures the matrix possesses certain desirable properties.
Key Properties of Positive Definite Matrices:
- Symmetry: All positive definite matrices are symmetric (A = A<sup>T</sup>). This symmetry is essential for many of their beneficial properties.
- Eigenvalues: As mentioned, all eigenvalues are strictly positive. This positivity is directly linked to the matrix's definiteness.
- Positive leading principal minors: The determinant of each leading principal submatrix is positive. This provides a practical way to check for positive definiteness.
- Cholesky Decomposition: Positive definite matrices can be uniquely decomposed into the product of a lower triangular matrix and its transpose (A = LL<sup>T</sup>). This decomposition is computationally efficient and crucial in various algorithms.
- Invertibility: All positive definite matrices are invertible. Their inverses are also positive definite.
Why are Positive Definite Matrices Important?
The importance of positive definite matrices stems from their implications for:
1. Optimization Problems:
Many optimization problems involve minimizing or maximizing a quadratic function. If the Hessian matrix (the matrix of second partial derivatives) of this function is positive definite, then the function has a unique global minimum. This ensures the solution found is optimal and not just a local minimum. This is critical in fields like:
- Machine Learning: Many machine learning algorithms, such as support vector machines (SVMs) and Gaussian processes, rely heavily on positive definite matrices (often called kernel matrices) to define similarity measures and solve optimization problems.
- Engineering: Optimal design problems, involving minimizing weight or maximizing strength, frequently involve positive definite matrices.
2. Statistics and Probability:
Positive definite matrices play a vital role in multivariate statistics. The covariance matrix of a multivariate random variable is always positive semi-definite (a generalization where eigenvalues can be zero). A positive definite covariance matrix indicates that the variables are not linearly dependent. This property is essential for:
- Principal Component Analysis (PCA): PCA utilizes the eigenvectors and eigenvalues of the covariance matrix (or correlation matrix) to reduce dimensionality. The positive definiteness guarantees the existence of real eigenvalues.
- Bayesian Inference: Many Bayesian methods utilize positive definite matrices to represent prior and posterior distributions.
3. Numerical Stability:
Algorithms that use positive definite matrices often exhibit superior numerical stability. The Cholesky decomposition, for example, provides a more stable way to solve linear systems than other methods like Gaussian elimination, especially when dealing with ill-conditioned matrices (matrices close to being singular).
Applications Across Disciplines:
Positive definite matrices appear in a wide variety of applications, including:
- Finite Element Analysis (FEA): In structural mechanics, the stiffness matrix is often positive definite.
- Image Processing: Positive definite matrices are used in image reconstruction and filtering.
- Quantum Mechanics: Density matrices, used to describe quantum systems, are positive semi-definite.
- Economics: Positive definite matrices are utilized in econometrics, particularly in modelling covariance structures.
Conclusion:
Positive definite matrices are fundamental mathematical objects with far-reaching consequences. Their properties guarantee robustness, efficiency, and stability in diverse applications. Understanding their characteristics is essential for anyone working with optimization, statistics, machine learning, or any field dealing with linear algebra and multivariate data. Their ubiquitous presence underscores their importance in building reliable and effective solutions for complex problems across numerous disciplines.
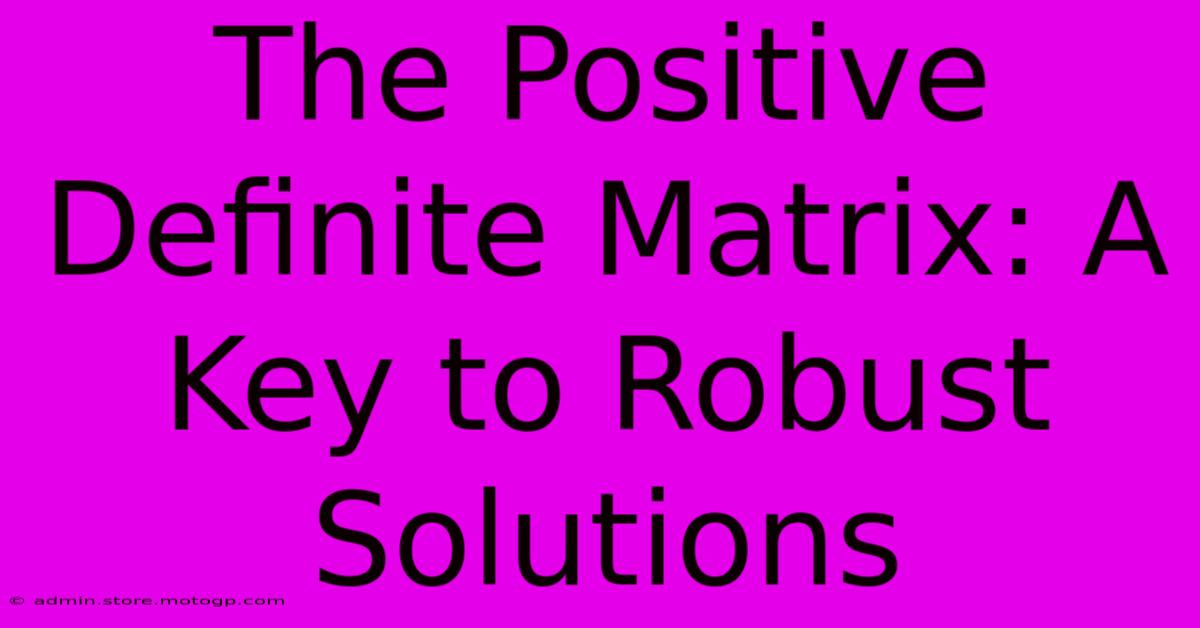
Thank you for visiting our website wich cover about The Positive Definite Matrix: A Key To Robust Solutions. We hope the information provided has been useful to you. Feel free to contact us if you have any questions or need further assistance. See you next time and dont miss to bookmark.
Featured Posts
-
Where Is The Mozambique Current And Why You Should Care
Feb 10, 2025
-
Unlocking The Sedan Car Type Explained
Feb 10, 2025
-
Beyond The Headlines The Real Story Of Najrans Women Martyrs
Feb 10, 2025
-
Wu Yi Fans Comeback Bigger And Better Than Ever
Feb 10, 2025
-
Confused About Buffs Huskers Stats Explained Simply
Feb 10, 2025